THE COMPANY WORDS KEEP: TRACING SEMANTIC CHANGE WITH DISTRIBUTIONAL METHODS
Patrícia Amaral (Joint work with Hai Hu and Sandra Kübler)
{pamaral,huhai,skuebler}@indiana.edu
Word meaning changes over time, a process that linguists call semantic change. The past few decades have seen an increase in research on semantic change, which can be considered “the last frontier” of linguistics; for a long time changes in the meaning of words were considered entirely idiosyncratic and hence not amenable to scientific study.
A relatively recent example of a change in word meaning comes from the word gay: in the early 1900s it meant ‘happy’ or ‘frolicsome’, as in example (1), while in (2) it denotes ‘homosexual’:
(1) She was a fine-looking woman, cheerful and gay (1900, Davies 2010)
(2) “I don’t personally support gay marriage myself”, Edwards said. (2000, Davies 2010)
Any speaker of English would probably concur with my judgement about (1) and (2).
And yet, how do we know that the meaning of gay has changed? How can we identify a change in word meaning without relying on our knowledge of the language?
One simple way to answer this question is literally to look around: to look at the neighbors of the word, i.e. the linguistic context preceding and following the word.
(1') a fine-looking woman, cheerful and gay
(2') I don’t personally support gay marriage
While in (1') we find adjectives like fine-looking and cheerful, in (2') the word gay is surrounded by the verb support and the noun marriage. If we looked at collocates of gay in recent data, we would indeed find that gay marriage is a highly frequent collocation.This simple example shows that change in context reveals change in meaning.
Linguists refer to this method of studying change through the “company words keep” (an expression originally used by Firth 1957) as distributional semantics. This change in neighbors of the word gay is visually represented in Figure 1, from Hamilton et al 2018. With very large amounts of data we can automatically examine how the neighbors of a word change in texts over time.
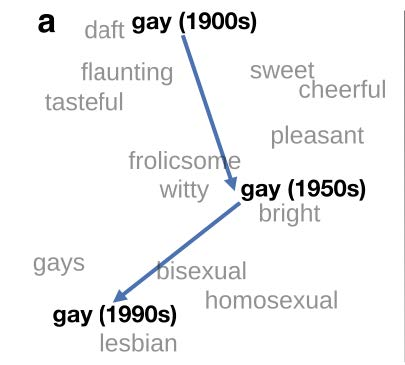
Figure 1: Visualization of the semantic change of the word gay through similar words using SGNS vectors, from Hamilton et al. 2018, Figure 1.
This type of approach captures the co-occurrence relationships of a word over time. In Figure 1, words are plotted in a vector space showing the change in the most frequent neighboring words in about a century.
If we can investigate changes in word distribution over time, we can investigate changes in word meaning.
This is the idea behind our project, which focuses specifically on using distributional methods to trace changes in meaning in Spanish. We want to start small. We begin with a pilot study comparing the results of a previous study on the semantic change of the word algo in Spanish (Amaral 2016). Since we are exploring novel methods, we first need to validate them. An intuition-based analysis of corpus data showed that in medieval texts algo meant ‘possessions, goods’ (as a noun) and ‘something’ (as an indefinite pronoun with inanimate reference), later acquiring the meaning of ‘a bit’ (i.e. a degree adverb). This later meaning is found since the end of the 15th century. Will distributional methods confirm this development or rather tell a different story? If the change is confirmed, will they provide us with new information to date this change?
But first things first. How do we find sentences containing the word to study in old texts? Where do we find our data?
Linguists studying language change rely on large digitized databases, like the Corpus Diacrónico del Español (CORDE) (http://corpus.rae.es/cordenet.html). In these databases we can search for the word of interest, as in Figure 2, using the website search tools. The results are called concordances:
Typically, historical linguistics would look at these lists of concordances and manually inspect them for the meaning of the sentence and the properties of neighboring words. This type of approach also targets the distribution of the word, but ultimately relies on the linguist’s intuition. Computational methods that use large amounts of data to automatically identify the co-occurrence statistics of a word do not depend on the linguist’s intuition or on human memory limitations. While word meaning has always been an elusive notion, tracking the combinatorial properties of a word over time is not only feasible but also objective and replicable.
Electronic databases like the CORDE do not let us access the texts freely: we can only see parts of the contexts and not the entire work. This is a big challenge, because computational methods require large amounts of data. We need freely downloadable historical texts from old stages of the language. For this reason, and with the invaluable help of the IDAH team, we have found another resource, the Digital Library of Old Spanish Texts, a project from the University of Wisconsin Madison. Within this resource, we have selected chronicle texts, a test set of ca. 7 million tokens. Texts from this digital library were extracted from the web.
As we obtained these files, we encountered the next roadblock: cleaning the data. Figure 3 below shows how the data looked like in the Hispanic Seminary website. These are transcriptions of paleographic editions of chronicles, containing annotations that describe e.g. column boundaries, the presence of illuminations, abbreviations used, variations in spelling or illegible text. The transcription manual explains all aspects of the faithful reproduction of the original texts. As important as this information is for scholars studying the transmission of texts through multiple editions, or the history of the book, for our purposes it means that the text is not ready for processing. We need plain text. In other words, we have to clean all these annotations and process the text in order to focus on neighboring words and nothing else.
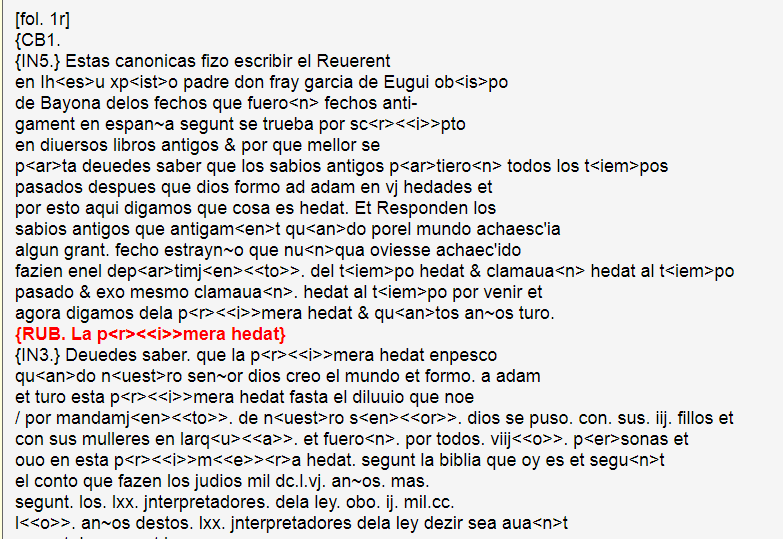
Manually cleaning these texts is not possible because it would take too long. On the other hand, automatic processing risks errors, like inadvertently splitting a word as two. In addition, there is loss of information: in the processed version we don’t know what comes from the original text and what was added/deleted/fixed by the transcribers. We also lose information regarding paragraph boundaries and sentence boundaries. When we clean the text automatically, we don’t know whether a dot means a period or something else (e.g. if it is used in numbering as in i. ii.). In fact, one common practice is to remove the punctuation altogether. But we get more normalized text, which allows computers to count the word occurrences.
The outcome of our preprocessing, with all punctuation removed and all letters lowercased, is shown in Figure 4:
As the description above makes apparent, this type of research requires a combination of people, skills, and time commitment that was only possible through the IDAH fellowship.
Once the text is at the stage displayed in Figure 4, we can actually start using the algorithms that track the distributional properties of algo. The (historical) linguist provides knowledge about the changes, the historical data and the texts, while the computational linguist contributes the tools to process the texts and automatically identify the combinatorial properties of the word. At every stage, we look at the data together to find the best solutions to analyze these specific texts.
We hope to be able to share our initial results soon! Stay tuned for the next steps of this project.